Much has been written on the need for statistics in genome-scale molecular biology. Very clever analytical approaches were devised, taking the form of carefully crafted and freely downloadable software packages. But still, every month or so, I meet with students and researchers facing a similar dilemma: they need to decide whether to report the strength of an effect (eg. gene X is over-expressed by 4.5-fold in condition A vs. B) or the significance of such effect (eg. gene X is overexpressed in condition A vs. B with a p-value of 0.0012).
A p-value is always linked to a statistical test which is nothing more than a mathematical formulation of a very precise question on the data. To derive a p-value, this question takes the form of an hypothesis (eg. gene X is not differentially expressed), called $H_0$ (null hypothesis) in the statistical jargon. A low p-value suggests that the hypothesis doesn’t hold (eg. the data does not support that gene X is not differentially expressed… notice the double negation!). In practice, this nuance bites in two cases: tiny, irrelevant differences in large datasets or solid differences accompanied with unexpected noise. Here are two simulated datasets (imagine qPCR quantification of a gene for samples under two conditions).
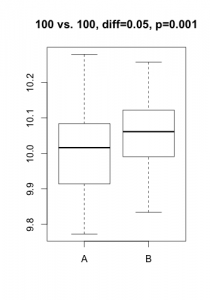
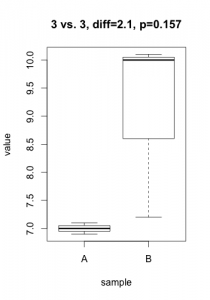
In the first scenario, the statistical test comes out significant even if the actual difference in expression is probably irrelevant (3% increase) and probably the result of some unwanted bias. In the second scenario, one of the 3 samples under condition ‘B’ misbehaved, resulting in a large difference becoming non-significant. For both cases, the average difference in expression would give a much better indication of what is going on. In most projects I’ve been involved in (microarrays, RNA-Seq, HTS, proteomics, qPCR, etc.), my first suggestion is always to start analyzing the results using strength of effect (differences, log-fold-change, %inhibition, etc.).
Then, what is the point of applying statistical tests? In what cases does it actually serve the underlying scientific question (does conditions A or B impact expression of gene X)? I’ll come back to this in a later post… but feel free to share your opinion in the meantime!
Bonjour,
Excellente discussion d’une question importante.
A quand la suite, pour répondre à la question du deuxième paragraphe?
Thanks Louis-Éric! [I’m taking the liberty to answer in English as you commented on this version of the text.] The follow-up post eventually morphed into http://bioinfo.iric.ca/tweaking-fishers-exact-test-for-biology/ . But, as I wrote this, I left out the idea that in most situations a confidence interval would best serve the purpose of assessing “biological significance” (in light of the variability, is this of interest) while acknowledging the continuous nature of the measure.